Open Journal of Mathematical Sciences
ISSN: 2523-0212 (Online) 2616-4906 (Print)
DOI: 10.30538/oms2022.0179
Optimal control strategy for the effectiveness of TB treatment taking into account the influence of HIV/AIDS and diabetes
Erick Manuel Delgado Moya\(^{1,*}\), Alain Pietrus\(^{2}\) and Sergio Muniz Oliva Filho\(^{1}\)
\(^{1}\) Institute of Mathematics and Statistics, Department of Applied Mathematics, University of Sao Paulo, Brazil.
\(^{2}\) University of Antilles, Department of Mathematics and Computer Sciences, LAMIA (EA 4540), BP 250, 97159, Pointe-a-Pitre, Guadeloupe, France.
Correspondence should be addressed to Erick Manuel Delgado Moya at erickmath@ime.usp.br
Abstract
Keywords:
1. Introduction
Tuberculosis is a chronic bacterial infectious disease caused by " Mycobacterium Tuberculosis". The main medical problems faced is the effectiveness of anti-TB treatments and extensively drug-resistant TB (XDR-TB) cause alarm of future non-effective TB drugs [1].
The number of cases of tuberculosis in the world has been increasing annually. For example in 2015, 10.4 million new cases were estimated in the world, 1.4 million TB-induced deaths, and 0.4 million deaths resulting from TB-HIV/AIDS co-infection [2].
The rifampcin, isoniazid, pyrazinamide, ethambtol are some of the first-line drugs for TB treatment and amikacin, capriomycim, cycloserine, azithromycin, clavithromycin, moxifloxacin, levofloxacin are the second line of treatment.
The treatment applied for active forms of tuberculosis is using first-line drugs (rifampcin, isoniazid, pyrazinamide, ethambtol) which belong to the first line of treatment for 4 months and followed by a daily intake of rifampcin and isoniazid for a period of four months.
Multidrug-resistant tuberculosis (MDR-TB) is caused by Mycobacterium tuberculosis strains with resistance to at least isoniazid and rifampin. Extensively drug-resistant tuberculosis (XDR-TB) is defined as a strain resistant to any type of fluoroquinolone and mainly to one of three injectable drugs: amikacin, capreomycin, or kanamycin in addition to isoniazid and rifampfin [3]. According to Gandhi et al., [4], about 3.2% of all new tuberculosis cases are multidrug-resistant (MDR-TB).
Diabetes is a risk factor for lower respiratory infections including TB and is a significant factor for TB infections at the population level [5]. Stevenson et al., reported that diabetes increases TB risk 1.5 to 7.8 times [6] and Jeon and Murray found that the relative risk for TB among diabetes patients was 3.11 [7]. Diabetes can affect the effectiveness of anti-TB drugs, especially rifampicin, by reducing their plasma concentrations [8]. Currently, the treatment regimen for diabetics and non-diabetics is the same [5].
TB can lead to impaired glucose tolerance (IGT) and new-onset diabetes [9]. In general, IGT normalizes once recovered from TB but remains an important risk factor for the future development of type 2 diabetes.
The rate of diabetes for HIV patients when they are infected is the same as for the general population. But certain metabolic factors related to HIV, and HIV therapy can increase the incidence of diabetes over 5 time. Cases of diabetes in HIV patients on antiretroviral therapy have been increasing. This problem appears to be related to the use of protease inhibitors and one of the solutions is the discontinuation of therapy. Patients on treatment for HIV should be monitored and consider to changes in therapy or specific treatment when metabolic problems occur.
Recurrent tuberculosis disease can occur as a result of reinfection, in which a patient becomes exogenously infected with a strain of Mycobacterium tuberculosis other than the organism that caused the original infection. A caveat in this regard is that, in high-incidence settings, patients, on rare occasions, may be exposed to or infected with a very similar or the same strain as in the primary infection, making it difficult to differentiate between relapse and reinfection in these particular cases [10]. Reinfection or reactivation is linked to the immune status of the patient and takes into account the behavior of the prevalence of the disease; in the case of HIV, the higher the prevalence, the higher the incidence of TB. Several studies have now shown that multiple genotypes can be detected by sampling both respiratory and extrapulmonary sites in seropositive individuals, illustrating the presence of migration routes within and between organs [11]. Reactivation TB may occur if the patient's immune system is weakened and cannot contain the latent bacteria. The bacteria then become active; they overload the immune system and cause the person to become ill with tuberculosis.
Optimal control theory has been used for studying the transmission dynamic of TB in [12,13,14,15,16]. Example, Kim et al., [12] proposed optimal control strategies to reduce the number of patients at high risk for latent and infectious tuberculosis with minimal intervention costs. Numerical simulation with data from the Philippines showed that distancing control is the most efficient control strategy when a single intervention is performed. Silva and Torres [13] applied optimal control theory using the prevention of exogenous reinfection as control in a tuberculosis disease. Jung et al., [14] applied optimal control theory to a two-strain tuberculosis model with aim to reduce the latent and infectious groups with the resistant-strain tuberculosis, where the controls are two types of treatments. Silva and Torres [16] applied optimal control theory to minimize the cost of interventions in a model of tuberculosis with reinfection and exposure.
The problems of HIV/AIDS control and TB-HIV/AIDS co-infection with different techniques have become a problem approached by researchers in last time. For example, Tahir et al., [17] extended a mathematical model of TB-HIV/AIDS co-infection to study the optimal control problem, and defined different schemes to minimize and control infection in any population. Awoke and Kassa [18] presented and studied a mathematical model for a transmission of TB-HIV/AIDS co-infection that incorporates prevalence dependent behaviour change in the population and treatment for the infected and applied optimal control theory to minimize the cost of infections and control enforcement efforts.
We can find applications of the optimal control theory in the study of TB-HIV/AIDS co-infection in [19,20,21,22]. Agusto and Adekunle [19] used optimal control theory to investigate optimal control strategies in disease spread and demonstrated that the combined strategy of preventing treatment failure in TB-infected individuals and treating individuals with drug-resistant TB is the most cost-effective. Silva and Torres [20] formulated a population model for TB-HIV/AIDS coinfection that considers antiretroviral therapy for HIV infection and treatments for latent and active TB, and used the theory of optimal control to reduce the number of individuals with active TB and AIDS. Fatmawati and Tasman [21] presented an optimal control problem on the treatment of the transmission of TB-HIV co-infection model, using anti-TB and antiretroviral treatments as controls. Tanvi and Aggarwal [22] presented an HIV/AIDS and TB co-infection model and proposed an optimal control problem to minimize the cost of the detection and treatment.
The study of diabetes control and its relationship to TB has increased in recent decades. For example, Kouidere et al., [23] proposed conducting awareness campaigns based on the severity of complications of diabetes, the importance of a balanced lifestyle, and correct use of treatment as an optimal control strategy. Chávez et al., [24] formulated a control system for optimal insulin delivery in type I diabetic patients using the linear and quadratic control problem theory. The linear model is used for the glucose-insulin dynamics and the non-linear for the evaluation of the regulatory controller.
The objective of this work is to present and solve the optimal control problem to reduce the resistance to tuberculosis treatment, taking into account the influence of HIV/AIDS and diabetes.
This paper is organized as follows: §2 briefly describes a mathematical model for the study of resistance to treatment of tuberculosis in the presence of HIV/AIDS and diabetes presented in [25]. In §3 is incorporated four controls in order to reduce the impact of TB treatment resistance and we study the optimal control problem. §4 includes numerical experimentation and §5 is about some conclusions.
2. Model formulation
In this section, we present the model with ordinary differential equations introduced in [25] and its basic properties. This model is designed for the study of MDR-TB and XDR-TB, taking into account the influence of HIV/AIDS and diabetes.The model compartments are: uninfected of TB, \(S_{T}\), \(S_{H}\), \(S_{D}\), latently infected, \(E_{T}\), \(E_{H}\), \(E_{D}\), individuals infected and drug-sensitive to TB (\(I_{T_{1}}\), \(I_{H_{1}}\), \(I_{D_{1}}\)), infected MDR-TB (\(I_{T_{2}}\), \(I_{H_{2}}\), \(I_{D_{2}}\)), infected XDR-TB (\(R_{T_{1}}\), \(R_{H_{1}}\), \(R_{D_{1}}\)) and recovered of TB (\(R_{T}\), \(R_{H}\), \(R_{D}\)) individuals where \(T\) represent TB-Only individuals, \(H\) are HIV/AIDS cases and \(D\) diabetics.
The \(M_{1}\), \(M_{2}\) and \(M_{3}\) are recruitment rates only-infected-TB, HIV/AIDS and diabetes respectively. The rate of acquiring diabetes by use of antiretroviral treatment is \(\alpha_{4}\) and the rate of an individual acquiring HIV and of developing diabetes are \(\alpha_{2}\) and \(\alpha_{1}\) respectively.
The TB infection rate is defined as \[\lambda_{T}=\alpha^{*}\dfrac{I_{T_{1}}+I_{T_{2}}+R_{T_{1}} +\epsilon_{1}( I_{H_{1}}+I_{H_{2}}+R_{H_{1}})+\epsilon_{2}( I_{D_{1}}+I_{D_{2}}+R_{D_{1}} )}{N}\] where \(\alpha^{*}\) is the effective contact rate and \(N\) is the total population. The parameters \(\epsilon_{j}\), \(j=1, 2\) are modifications parameters, modeling the increased infectiousness in HIV/AIDS and diabetics. The natural death rate \(\mu\) is the same from any compartment and \(u_{11}\) and \(u_{12}\) are death rate associated with HIV/AIDS and diabetes. The \(\eta\) is defined as the natural rate of progression of tuberculosis. The \(\beta^{*}\) represents the proportion of active TB cases that are resistant. The \(\omega_{1}\), \(\omega_{2}\), are the parameters associated with the transmission of tuberculosis in the HIV/AIDS and diabetes compartments where \(\omega_{1}, \omega_{2}>1\).
We assume that TB-recovered \(R_{i}\), \(i= T,D,H\) acquire partial immunity so that from the recovered compartment (the cases that recover) enter the latent compartment with a parameter associated with TB reinfection and reactivation \(\beta^{'}_{1}\) with \(\beta^{'}_{1}\leq 1\).
We define \(\epsilon^{*}_{j}\), \(j=1,2\) as the parameters modification associated with resistance to tuberculosis treatment in HIV/AIDS and diabetics.
The \(t_{1}\) and \(t_{2}\) are modifications parameters associated with diabetes or HIV infection from the compartments of active TB infection. We define death from TB with a rate \(d_{1}\), deaths from the combination TB and HIV/AIDS with a rate \(d_{2}\) and deaths from the combination TB and diabetes with a rate \(d_{3}\) and we assumed that \(d_{3} \geq d_{1}\) and \(d_{2} \geq d_{1}\) as diabetes and HIV/AIDS experience greater disease induced deaths than their corresponding only TB and we assume death from TB after the use of treatment. The rates \(l_{1}\), \(l_{2}\) and \(l_{3}\) represent the cases that will be MDR-TB (first resistance). The \(p_{1}\), \(p_{2}\) and \(p_{3}\) represent the rates related to developing XDR-TB. The \(t_{3}\) parameter is associated with the combination of antirretroviral therapy and the treatment of tuberculosis and the possibility of developing diabetes. The \(\eta_{11}\), \(\eta_{12}\) and \(\eta_{13}\) is the recovery rate after being sensitive TB and \(m_{1}\), \(m_{2}\) and \(m_{3}\) is the recovery rate after being MDR-TB. Let's assume that \(\eta_{1l}> m_{l}\) for \(l=1,2,3\). The \(t^{'}_{l}, l=1,2,3\) are modification parameters associated with TB deaths in MDR-TB cases.
The \(\eta^{*}_{11}\), \(\eta^{*}_{12}\) and \(\eta^{*}_{13}\) are the recovery rate after being XDR-TB. Let's assume that \(\eta_{1l}> \eta^{*}_{1l}\) and \(m_{l}>\eta^{*}_{1l}\) for \(l=1,2,3\). The \(t^{*}_{1}\), \(t^{*}_{2}\) and \(t^{*}_{3}\) are modification parameters associated with death by TB, death by combination TB-HIV/AIDS and by combination TB-Diabetes after being XDR-TB.
The variables and parameters of the model are represented in the Table 1.
Table 1. Variables and parameters of model.
Parameter or Variable | Description |
---|---|
\(S_{T}\), \(S_{H}\), \(S_{D}\) | Uninfected of TB |
\(E_{T}\), \(E_{H}\), \(E_{D}\) | Latently infected |
\(I_{T_{1}}\), \(I_{H_{1}}\), \(I_{D_{1}}\) | Drug-sensitive TB |
\(I_{T_{2}}\), \(I_{H_{2}}\), \(I_{D_{2}}\) | Infected MDR-TB |
\(R_{T_{1}}\), \(R_{H_{1}}\), \(R_{D_{1}}\) | Infected XDR-TB |
\(R_{T}\), \(R_{H}\), \(R_{D}\) | Recovered of TB |
\(M_{1},\) \(M_{2},\) \(M_{3}\) | Recruitment rates |
\(\alpha^{*}\) | Effective contact rates for TB infection |
\(\alpha_{1}\) | Acquiring diabetes rate |
\(\alpha_{2}\) | Acquiring HIV rate |
\(\alpha_{4}\) | Diabetes development rate by use of HIV therapy |
\(\omega_{1},\) \(\omega_{2},\) \(\epsilon_{1}, \epsilon_{2}\) | Modification parameters |
\(\mu\) | Natural mortality rate |
\(\eta\) | Natural rate of progression to active TB |
\(t_{1}, t_{2}, t_{3}, t^{*}_{1}, t^{*}_{2}, t^{*}_{3}\) | Modification parameters |
\(t^{'}_{1}, t^{'}_{2}, t^{'}_{3}\) | Modification parameters |
\(\epsilon^{*}_{1},\epsilon^{*}_{2},\) \(\beta^{'}_{1}\) | Modification parameters |
\(l_{1},\) \(l_{2},\) \(l_{3}\) | Resistant TB development rates |
\(d_{1}\) | TB induced death rate |
\(d_{2}\) | TB-HIV induced death rate |
\(d_{3}\) | TB-Diabetes induced death rate |
\(\mu_{11}, \mu_{12}\) | Death rate of HIV/AIDS and diabetes respectively |
\(m_{1},\) \(m_{2},\) \(m_{3}\) | TB recovery rates for MDR-TB |
\(\beta^{*}\) | Proportion of active TB cases that are resistant. |
\(\eta_{11},\) \(\eta_{12},\) \(\eta_{13}\) | TB recovery rates of drug-sensitive TB infected |
\(\eta_{14},\) \(\eta_{15},\) \(\eta_{16}\) | Resistant (XDR-TB) TB development rates after being MDR-TB |
\(\eta_{11}^{*},\) \(\eta_{12}^{*},\) \(\eta_{13}^{*}\) | TB recovery rates of XDR-TB |
\(p_{1}, p_{2}, p_{3}\) | Rates related to developing XDR-TB |
The effectiveness of the TB treatment with the presence of HIV/AIDS and diabetes is modeled with the following system of differential equations:
The following results present the existence and positivity of the solution of the system (1) and the region where all variables are always non-negative that is defined as a biologically feasible region. These results and their proof are presented in [25].
Theorem 1. Let the initial data for the model (1) be \(S_{i}(0)> 0, E_{i}(0)> 0, I_{i_{1}}(0) > 0, I_{i_{2}}(0)> 0, R_{i_{1}}(0)> 0, R_{i}(0)> 0, i=T, H, D.\) Then the solutions \((S_{i}(t), E_{i}(t), I_{i_{1}}(t), I_{i_{2}}(t), R_{i_{1}}(t), R_{i}(t)), i=T, H, D\) of the model (1), with positive initial data, will remain positive for all time \(t> 0.\)
Theorem 2. The solutions of model system (1) with non-negative initial conditions exist for all times.
Lemma 1. The closed set \[\Omega= \bigg\lbrace (S_{i}, E_{i}, I_{i_{1}}, I_{i_{2}}, R_{i_{1}}, R_{i})\in \mathbb{R}^{18}_{+}, i= T,H, D:N(t) \leq \dfrac{M_{1}+M_{2}+M_{3}}{\mu}\bigg\rbrace \] is positively-invariant and attracts all positive solutions of the model (1).
The model (1) is mathematically and epidemiologically well posed in \(\Omega\) which is the biologically feasible region.The basic reproduction number is found for the different sub-populations (TB-HIV/AIDS, TB-Diabetes and TB-Only) in order to study the transmission of tuberculosis in these sub-populations, using the next generation matrix theory [26]. The basic reproduction numbers and the results relating the stability at the disease-free equilibrium points with the basic reproduction number are given in [25].
The basic reproduction number for the sub-model where we study cases without the presence of HIV/AIDS and diabetes (TB-Only) (\(S_{H}= S_{D}=E_{H}=E_{D}= I_{H_{1}}= I_{H_{2}}= I_{D_{1}}=I_{D_{2}}=R_{H_{1}}=R_{D_{1}}= R_{H}= R_{D}=0\)) is defined as
Lemma 2. The disease-free equilibrium point \(\epsilon_{0}^{T}\) is locally asymptotically stable when \( \Re_{0}^{T}< 1\) and unstable when \( \Re_{0}^{T}> 1.\)
The basic reproduction number for the sub-model where we study sub-population of TB-HIV/AIDS (\(S_{D}=S_{T}=E_{D}=E_{T}=I_{D_{1}}=I_{D_{2}}=I_{T_{1}}=I_{T_{2}}=R_{T_{1}}=R_{T}=R_{D_{1}}=R_{D}=0\)) isLemma 3. The disease-free equilibrium \(\epsilon_{0}^{H}\) is asymptotically stable when \( \Re^{H}_{0}< 1\) and is unstable whenever \( \Re^{H}_{0}> 1.\)
The basic reproduction number for the TB-Diabetes sub-population (\(S_{H}=S_{T}=E_{H}=E_{T}=I_{H_{1}}=I_{H_{2}}=I_{T_{1}}=I_{T_{2}}=R_{H_{1}}=R_{H}=R_{T_{1}}=R_{T}=0\)) isLemma 4. The disease-free equilibrium \(\epsilon_{0}^{D}\) is asymptotically stable when \( \Re^{D}_{0}< 1\) and is unstable whenever \( \Re^{D}_{0}> 1.\)
For the full model (1), infection-free equilibrium point is \[\epsilon_{0}^{G}= (S^{T}_{0}, S^{H}_{0}, S^{D}_{0}, 0, 0, 0, 0, 0, 0, 0, 0, 0, 0, 0, 0, 0, 0, 0)\] and the dominant eigenvalues of the Jacobian of the full system (1) in \(\epsilon_{0}^{G}\) are \(\Re_{0}^{T},\Re_{0}^{H}\) and \(\Re_{0}^{D}\). Then, the basic reproduction number of the model (1) is \[\Re_{0}= \max \lbrace \Re_{0}^{T},\Re_{0}^{H}, \Re_{0}^{D} \rbrace.\]2.1. Global stability
Now, we list two conditions that if, also guarantee the global asymptotic stability of the disease-free equilibrium point. Following [27], we can rewrite the model (1) asThe disease-free equilibrium is now denoted by \(E_{0}^{G}= (S^{*}_{0}, 0)\) where \(S^{*}_{0}=(S_{0}, 0,0,0)\) and \(S_{0}=(S^{T}_{0},S^{H}_{0}, S^{D}_{0})\), \(S^{T}_{0}= \bigg(\dfrac{M_{1}}{\mu+\alpha_{1}+\alpha_{2}},0 \bigg)\), \(S^{H}_{0}= \bigg(\dfrac{M_{2}}{\mu+\mu_{11}+\alpha_{4}},0 \bigg)\) and \(S^{D}_{0}= \bigg(\dfrac{M_{3}}{\mu+\mu_{12}+\alpha_{2}},0 \bigg)\).
The conditions \(H_{1}\) and \(H_{2}\) below must be satisfied to guarantee the global asymptotic stability of \(E_{0}^{G}\).
If model (1) satisfies the conditions \(H_{1}\) and \(H_{2},\) then the following results holds;
Theorem 3. The fixed point \(E_{0}^{G}\) is a globally asymptotically stable equilibrium of model (1) provided that \(\Re_{0}< 1\) and that the conditions \(H_{1}\) and \(H_{2}\) are satisfied.
Proof. Let \[F(S, 0)= \begin{pmatrix} M_{1}-(\mu +\alpha_{1}+\alpha_{2}) S_{T} \\ M_{2}-(\mu +\mu_{11}+\alpha_{4}) S_{H} \\ M_{3}-(\mu+\mu_{12} +\alpha_{1}) S_{D} \\ 0\\ 0\\ 0 \end{pmatrix}.\] As \(F(S, 0)\) is a linear equation, we have that \(S^{*}_{0}\) is globally stable, hence \(H_{1}\) is satisfied. Then, \begin{align*} \mathbf{A}&={\tiny\left(\begin{array}{cccccccccccc} -k_{11} & 0 &0& \alpha^{*}& \alpha^{*} & \alpha^{*}\epsilon_{1} & \alpha^{*}\epsilon_{1}& \alpha^{*}\epsilon_{2} & \alpha^{*}\epsilon_{2} &\alpha^{*}& \alpha^{*}\epsilon_{1} & \alpha^{*}\epsilon_{2}\\ \alpha_{2} &-k_{21} &\alpha_{2}& \omega_{1}\alpha^{*}& \omega_{1}\alpha^{*} & \omega_{1}\alpha^{*}\epsilon_{1} & \omega_{1}\alpha^{*}\epsilon_{1}& \omega_{1}\alpha^{*}\epsilon_{2} & \omega_{1}\alpha^{*}\epsilon_{2}&\omega_{1}\alpha^{*}& \omega_{1}\alpha^{*}\epsilon_{1} & \omega_{1}\alpha^{*}\epsilon_{2} \\ \alpha_{1} &\alpha_{4} &-k_{31}& \omega_{2}\alpha^{*}& \omega_{2}\alpha^{*} & \omega_{2}\alpha^{*}\epsilon_{1} & \omega_{2}\alpha^{*}\epsilon_{1}& \omega_{2}\alpha^{*}\epsilon_{2} & \omega_{2}\alpha^{*}\epsilon_{2} &\omega_{2}\alpha^{*}& \omega_{2}\alpha^{*}\epsilon_{1} & \omega_{2}\alpha^{*}\epsilon_{2}\\ (1-\beta^{*})\eta & 0 & 0& -k_{12} & 0&0 &0 &0& 0&0 &0 &0 \\ (1-p_{1})\beta^{*}\eta & 0 & 0& l_{1} & -k_{13}&0 &0 &0& 0&0 &0 &0 \\ 0& (1-\beta^{*})\epsilon^{*}_{1}\eta & 0&\alpha_{2} & 0&-k_{22} &\alpha_{2} &0& 0&0 &0 &0 \\ 0& (1-P_{2})\beta^{*}\epsilon^{*}_{1}\eta & 0& 0&\alpha_{2} &l_{2} &-k_{23} &0& \alpha_{2}&0 &0 &0 \\ 0& 0&(1-\beta^{*})\epsilon^{*}_{2}\eta &\alpha_{1} & 0&\alpha_{4} &0 &-k_{32}& 0&0 &0 &0 \\ 0& 0&(1-p_{3})\beta^{*}\epsilon^{*}_{2}\eta &0 & \alpha_{1}&0 &\alpha_{4} &l_{3}& -k_{33}&0 &0 &0 \\ p_{1}\beta^{*}\eta & 0 & 0 &0 & \eta_{14}& 0& 0&0 & 0&-k_{14} &0 &0\\ 0& p_{2}\epsilon^{*}_{1}\beta^{*}\eta & 0 &0 & 0& 0& \eta_{15}&0 & 0&\alpha_{2} &-k_{24} &\alpha_{2}\\ 0& 0 & p_{3}\epsilon^{*}_{2}\beta^{*}\eta &0 & 0& 0& 0&0 & \eta_{16}&\alpha_{1} &\alpha_{4} &-k_{34}\\ \end{array}\right)},\\ \mathbf{I}&=\left(\begin{array}{cccccccccccc} E_{T}, &E_{H}, & E_{D}, & I_{T_{1}}, & I_{T_{2}}, & I_{H_{1}}, & I_{H_{2}}, & I_{D_{1}}, & I_{D_{2}}, & R_{T_{1}}, & R_{H_{1}}, & R_{D_{1}} \\ \end{array}\right),\\ G^{*}(S,I)&=AI^{T}-G(S,I),\\ G^{*}(S,I)&={\small\begin{pmatrix} G^{*}_{1}(S,I) \\ G^{*}_{2}(S,I)\\ G^{*}_{3}(S,I)\\ G^{*}_{4}(S,I)\\ G^{*}_{5}(S,I) \\ G^{*}_{6}(S,I)\\ G^{*}_{7}(S,I)\\ G^{*}_{8}(S,I)\\ G^{*}_{9}(S,I) \\ G^{*}_{10}(S,I)\\ G^{*}_{11}(S,I)\\ G^{*}_{12}(S,I) \end{pmatrix}=\begin{pmatrix} \alpha^{*}(I_{T_{1}}+I_{T_{2}}+R_{T_{1}}+\epsilon_{1}({I_{H_{1}}+I_{H_{2}}+R_{H_{1}}})+\epsilon_{2}(I_{D_{1}}+I_{D_{2}}+R_{D_{1}}))\bigg( 1- \dfrac{S_{T}+\beta^{'}_{1}R_{T}}{N}\bigg) \\ \omega_{1}\alpha^{*}(I_{T_{1}}+I_{T_{2}}+R_{T_{1}}+\epsilon_{1}({I_{H_{1}}+I_{H_{2}}+R_{H_{1}}})+\epsilon_{2}(I_{D_{1}}+I_{D_{2}}+R_{D_{1}}))\bigg( 1- \dfrac{S_{H}+\beta^{'}_{1}R_{H}}{N}\bigg) \\ \omega_{2}\alpha^{*}(I_{T_{1}}+I_{T_{2}}+R_{T_{1}}+\epsilon_{1}({I_{H_{1}}+I_{H_{2}}+R_{H_{1}}})+\epsilon_{2}(I_{D_{1}}+I_{D_{2}}+R_{D_{1}}))\bigg( 1- \dfrac{S_{D}+\beta^{'}_{1}R_{D}}{N}\bigg) \\ 0\\ 0\\ 0\\ 0\\ 0\\ 0\\ 0\\ 0\\ 0 \end{pmatrix}}. \end{align*} Since \(S_{T}+\beta^{'}_{1}R_{T}\), \(S_{H}+\beta^{'}_{1}R_{H}\) and \(S_{D}+\beta^{'}_{1}R_{D}\) are always less than or equal to \(N_{T},\) \(\dfrac{S_{T}+\beta^{'}_{1}R_{T}}{N}\leq 1\), \(\dfrac{S_{H}+\beta^{'}_{1}R_{H}}{N}\leq 1\) and \(\dfrac{S_{D}+\beta^{'}_{1}R_{D}}{N}\leq 1.\) Thus \(G^{*}(S,I) \geq 0\) for all \((S,I) \in D.\) The \(E_{0}^{G}\) is a globally asymptotically stable.
3. Model with controls and optimal control problem
3.1. Definition of controls and its policy
Our aim with the help of the control optimal theory is to decrease the total number of patients with MDR-TB and XDR-TB during a period of time \([t_{0},t_{f}]\). The control strategy is decomposed in four controls \(u_{0}\), \(u_{11}\), \(u_{12}\) and \(u_{13}\) defined as follows:- \(u_{0}(t)\) (control over reinfection and reactivation)- this refers to preparing patients recovering from TB to avoid possible reinfection or reactivation of the bacteria, scheduling medical consultations, and lab tests periodically. Control of entry of new genotypes of TB into the population. Also, to inform patients how to maintain an active immune system, particularly immunocompromised patients (HIV/AIDS) with adherence to antiretroviral treatment, stimulation of a good (healthy) diet, physical exercise, among others.
- \(u_{11}(t)\) (control for TB-Only)- this includes personal respiratory protection, educational programs for public health, activities that ensure treatment completion to reduce relapse following treatment. Patients receiving treatment for MDR-TB should be monitored to ensure the completion of the treatment. As part of this control, it is needed to check blood glucose levels and make HIV tests to determine if the person is diabetic and/or HIV positive.
- \(u_{12}(t)\) (control for HIV/AIDS cases)- the control will be based on clinical follow-up (we assume all cases are diagnosed), and we consider all cases are using antiretroviral therapy and have a follow-up on their CD4 count and viral load. In particular, from the beginning of treatment for TB, the return of the patient should occur in up to 15 days. Monthly consultations until the end of the TB treatment. Consultations by other members of the multi-professional team, with the objective of promoting adherence to treatment, identifying interoccurrences that may interfere with the correct use of TB drugs and antiretrovirals. Another important element is to check blood glucose levels and determine if the person is diabetic.
- \(u_{13}(t)\) (control for diabetics cases)- the control is focused on monitoring glycemic parameters throughout TB treatment, and promoting adherence to treatment, identifying interoccurrences that may interfere with the efficacy of TB treatment. Another important factor is to make HIV tests to control the exits of this subpopulation.
In particular, \(u_{11}(t)\) is the control in the entrance to compartment \(I_{T_{2}}\), \(R_{T_{1}}\), \(u_{12}(t)\) is the control in the entrance to compartment \(I_{H_{2}}\), \(R_{H_{1}}\) and \(u_{13}(t)\) is the control in the entrance to compartment \(I_{D_{2}}\), \(R_{D_{1}}\). The \(u_{0}(t)\) controls entry into the \(E_{T}\), \(E_{H}\) and \(E_{D}\) compartments by reinfection or reactivation. Eqs (7) shows the incorporation of the controls in the compartments of model (1) and the other equations remain the same as the uncontrolled model (1). The \((1-u_{0})\), \((1-u_{11})\), \((1-u_{12})\) and \((1-u_{13})\) representing effort that prevents failure of the treatment. The Figure 1 shows the control dynamics.
Figure 1. Schematic representation of model with controls, the arrows (discontinued) and boxes red represents the inputs and compartments to be controlled.
3.2. Optimal control problem and its analysis
Our objective functional to be minimized is \begin{align*} J(u_{0},u_{11}, u_{12}, u_{13})= & \int^{t_{f}}_{t_{0}} (E_{T}(t)+E_{H}(t)+E_{D}(t))+(I_{T_{2}}(t)+I_{H_{2}}(t)+I_{D_{2}}(t))+(R_{T_{1}}(t)+R_{H_{1}}(t)+R_{D_{1}}(t))\\ & +\dfrac{1}{2}\bigg(B_{0}u^{2}_{0}(t)+(B_{1}+B_{4})u_{11}^{2}(t)+(B_{2}+B_{5})u_{12}^{2}(t)+(B_{3}+B_{6})u_{13}^{2}(t)\bigg)dt. \end{align*} The structure of our functional is consistent with recent works as [12,13,14,15,16,17,18,19].The coefficients \(B_{j}, j = 0,1,...,6\) represent the constant weight associated with the relative costs of implementing the respective control strategies on a finite time horizon \([t_{0}, t_{f}]\) (were initial time \(t_{0}=0\), final time \(t_{f}=10\) year period) and consists in the cost induced by the efforts of the three different types of control. The \(B_{1}\), \(B_{2}\) and \(B_{3}\) are associated with the implementation of control on the MDR-TB and \(B_{4}\), \(B_{5}\) and \(B_{6}\) to the XDR-TB. Given the characteristics of resistance to tuberculosis and its treatment, which in some cases may include hospitalization, high drug costs, the use of other drugs to stimulate the immune system, among others, let's assume that \(B_{1}< B_{4}\), \(B_{2}< B_{5}\) and \(B_{3}< B_{6}\) and these constants cannot be neither zeros nor very large (realistic values). Given the characteristics of resistance to tuberculosis and its treatment, which in some cases may include hospitalization, high drug costs, the use of other drugs to stimulate the immune system, among others, let's assume that \(B_{1}< B_{4}\), \(B_{2}< B_{5}\) and \(B_{3}< B_{6}\) and these constants cannot be neither zeros nor very large (realistic values).
The cost involved in the control about the compartments \(I_{T_{2}}\), \(I_{H_{2}}\) and \(I_{D_{2}}\) is taken as \(\int^{t_{f}}_{t_{0}}\frac{B_{1}u^{2}_{11}}{2}\), \(\int^{t_{f}}_{t_{0}}\frac{B_{2}u^{2}_{12}}{2}\), \(\int^{t_{f}}_{t_{0}}\frac{B_{3}u^{2}_{13}}{2}\), for \(R_{T_{1}}\), \(R_{H_{1}}\), \(R_{D_{1}}\) are \(\int^{t_{f}}_{t_{0}}\frac{B_{4}u^{2}_{11}}{2}\), \(\int^{t_{f}}_{t_{0}}\frac{B_{5}u^{2}_{12}}{2}\), \(\int^{t_{f}}_{t_{0}}\frac{B_{6}u^{2}_{13}}{2}\), for \(E_{T}\), \(E_{H}\) and \(E_{D}\) are \(\int^{t_{f}}_{t_{0}}\frac{B_{0}u^{2}_{0}}{2}\). We seek to find the optimal controls \(u^{*}_{0}\), \(u^{*}_{11}\), \(u^{*}_{0}\), \(u^{*}_{12}\) and \(u^{*}_{13}\) that satisfy
3.2.1. The necessary and sufficient conditions of optimal control
We will study the sufficient conditions for the existence of an optimal control for our controls system using the conditions in Theorem 4.1 and its corresponding Corollary in [28]. After that, we will characterize the optimal control functions by using Pontryagin’s Maximum Principle and then we derive necessary conditions for our control problem. We have that solutions of the controls system are bounded and non-negative for finite time interval in the biologically feasible region. These results are important to establish the existence of an optimal control.We denote the vector of states \(\vec{x}=[S_{T}, S_{H}, S_{D}, E_{T}, E_{H}, E_{D}, I_{T_{1}}, I_{T_{2}}, I_{H_{1}},I_{H_{2}}, I_{D_{1}}, I_{D_{2}}, R_{T_{1}}, R_{H_{1}}, R_{D_{1}}, R_{T}, R_{H}, R_{D}]^{T}\) and the controls vector \(\vec{u}=[u_{0},u_{11}, u_{12}, u_{13}]^{T}\).
Theorem 4. There exists an optimal control \(u^{*}=(u^{*}_{0}, u^{*}_{11}, u^{*}_{12}, u^{*}_{13})\) to problem \[\min J(u_{0}, u_{11}, u_{12}, u_{13}) \quad \text{subject to model (1) with controls}\] where \(u^{*}\in U_{ad}.\)
Proof. We use the requirements of Theorem 4.1 and Corollary 4.2 in [28] to prove the Theorem 4. Let \(l(t, \vec{x}, \vec{u})\) as the right-hand of (1) with controls. We will show that the following requirements are satisfied:
- I. \(l\) is of class \(C^{1}\) and there exist a constant \(C\) such that
\(\vert l(t,0,0)\vert \leq C\), \(\vert l_{x}(t, \vec{x}, \vec{u})\vert \leq C(1+\vert \vec{u}\vert)\), \(\vert l_{u}(t, \vec{x}, \vec{u}) \vert \leq C\).
- II. The admissible set \(\mathbb{F}\) of all solution to system (1) with controls in \(U_{ad}\) is non empty;
- III. \(l(t, \vec{x}, \vec{u})= a_{1}(t, \vec{x})+a_{2}(t, \vec{x})\vec{u};\)
- IV. The control set \(U= [0,1]\times [0, 1]\times[0, 1]\) is closed, convex and compact;
- V. The integrand of the objective functional is convex in \(U\).
By the construction of the model and the condition I., system (1) with controls has a unique solution for constant controls, this implies that condition II. is satisfied.
We can write the system (1) with controls as
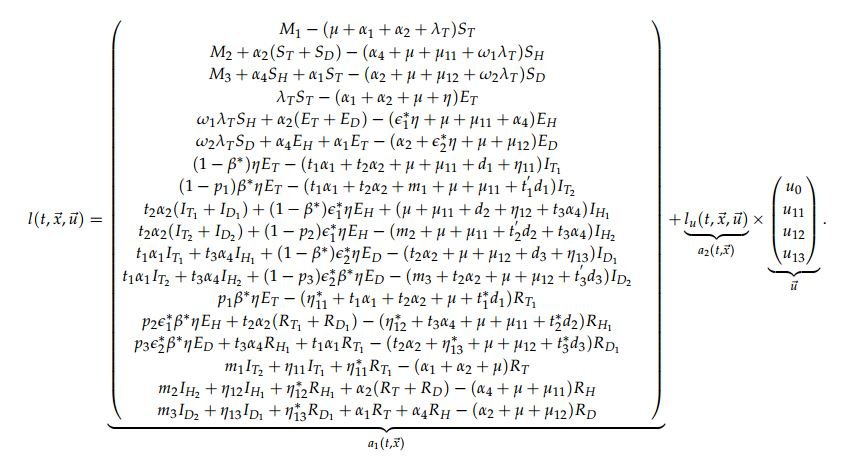
This means that condition III. holds. By construction the sets \(U\) is closed, convex and compact and condition IV. is satisfied.
Now, we are going to prove the convexity of the integrand in the objective functional
\begin{align*} \label{h1} f(t, \vec{x}, \vec{u})=&E_{T}(t)+E_{H}(t)+E_{D}(t)+I_{T_{2}}(t)+I_{H_{2}}(t)+I_{D_{2}}(t)+I_{T_{3}}(t)+I_{H_{3}}(t)+I_{D_{3}}(t)+ \dfrac{B_{0}u^{2}_{0}(t)}{2}\\ \nonumber &+\dfrac{(B_{1}+B_{4})u_{11}^{2}(t)}{2}+\dfrac{(B_{2}+B_{5})u_{12}^{2}(t)}{2}+\dfrac{(B_{3}+B_{6})u_{13}^{2}(t)}{2}, \end{align*} this implies proving that \[(1-q)f(t, \vec{x}, \vec{u})+qf(t, \vec{x}, \vec{v}) \geq f(t, \vec{x}, (1-q)\vec{u}+q\vec{v}),\] where \(\vec{u}\), \(\vec{v}\) are two control vectors with \(q\in [0,1]\). It follows that \begin{align*} &(1-q)f(t, \vec{x}, \vec{u})+qf(t, \vec{x}, \vec{v})= (1-q)\bigg(E_{T}+E_{H}+E_{D}+I_{T_{2}}+I_{H_{2}}+I_{D_{2}}+I_{T_{3}}+I_{H_{3}}+I_{D_{3}}+\dfrac{B_{0}u^{2}_{0}}{2}+\dfrac{(B_{1}+B_{4})u_{11}^{2}}{2}\\ &+\dfrac{(B_{2}+B_{5})u_{12}^{2}}{2}+ \dfrac{(B_{3}+B_{6})u_{13}^{2}}{2}\bigg) +q\bigg(E_{T}+E_{H}+E_{D}+I_{T_{2}}+I_{H_{2}}+I_{D_{2}}+I_{T_{3}}+I_{H_{3}}+I_{D_{3}} +\dfrac{B_{0}v^{2}_{0}}{2}+\dfrac{(B_{1}+B_{4})v_{11}^{2}}{2}\\ &+\dfrac{(B_{2}+B_{5})v_{12}^{2}}{2}+ \dfrac{(B_{3}+B_{6})v_{13}^{2}}{2}\bigg)\\ &= E_{T}+E_{H}+E_{D}+I_{T_{2}}+I_{H_{2}}+I_{D_{2}}+I_{T_{3}}+I_{H_{3}}+I_{D_{3}}+\bigg( \dfrac{B_{0}(qv^{2}_{0}+(1-q)u^{2}_{0})}{2}+ \dfrac{(B_{1}+B_{4})(qv_{11}^{2}+(1-q)u_{11}^{2})}{2}\\ &+ \dfrac{(B_{2}+B_{5})(qv_{12}^{2}+(1-q)u_{12}^{2})}{2}+\dfrac{(B_{3}+B_{6})(qv_{13}^{2}+(1-q)u_{13}^{2})}{2}\bigg) \end{align*} and \begin{align*} & f(t, \vec{x}, (1-q)\vec{u}+q\vec{v})= (E_{T}+E_{H}+E_{D}+I_{T_{2}}+I_{H_{2}}+I_{D_{2}}+I_{T_{3}}+I_{H_{3}}+I_{D_{3}}+\dfrac{B_{0}}{2}\bigg[(1-q)u_{0}+qv_{0}\bigg]^{2}\\ &+\dfrac{(B_{1}+B_{4})}{2}\bigg[(1-q)u_{11}+qv_{11}\bigg]^{2}+\dfrac{(B_{2}+B_{5})}{2}\bigg[(1-q)u_{12}+qv_{12}\bigg]^{2}+\dfrac{(B_{3}+B_{6})}{2}\bigg[(1-q)u_{13}+qv_{13}\bigg]^{2}. \end{align*} Then, we have \begin{align*} &(1-q)f(t, \vec{x}, \vec{u})+qf(t, \vec{x}, \vec{v})-f(t, \vec{x}, (1-q)\vec{u}+q\vec{v})\\ &= \dfrac{B_{0}}{2}\bigg((1-q)u^{2}_{0}+ q v^{2}_{0}-((1-q)u_{0}+qv_{0})^{2}\bigg)+ \dfrac{(B_{1}+B_{4})}{2}\bigg((1-q)u^{2}_{11}+ q v^{2}_{11}-((1-q)u_{11}+qv_{11})^{2}\bigg)\\ &+\dfrac{(B_{2}+B_{5})}{2}\bigg((1-q)u^{2}_{12}+ q v^{2}_{12}-((1-q)u_{12}+qv_{12})^{2}\bigg)+\dfrac{(B_{3}+B_{6})}{2}\bigg((1-q)u^{2}_{13}+ q v^{2}_{13}-((1-q)u_{13}+qv_{13})^{2}\bigg)\\ &=\dfrac{B_{0}}{2}\bigg[\sqrt{q(1-q)}u_{0}-\sqrt{q(1-q)}v_{0}\bigg]^{2}+ \dfrac{(B_{1}+B_{4})}{2}\bigg[\sqrt{q(1-q)}u_{11}-\sqrt{q(1-q)}v_{11}\bigg]^{2}\\ &+\dfrac{(B_{2}+B_{5})}{2}\bigg[\sqrt{q(1-q)}u_{12}-\sqrt{q(1-q)}v_{12}\bigg]^{2}+ \dfrac{(B_{3}+B_{6})}{2}\bigg[\sqrt{q(1-q)}u_{13}-\sqrt{q(1-q)}v_{13}\bigg]^{2}\geq 0. \end{align*} With this, we prove the requirement V. and the proof of the theorem is complete. The Pontryagin's Maximum Principle, provides the necessary conditions an optimal control must satisfy. Firstly, the Hamiltonian for the control problem is defined byTheorem 5. Given an optimal controls \(u^{*}_{0}, u^{*}_{11}\), \(u^{*}_{12}\), \(u^{*}_{13}\) and associated solutions \(S^{*}_{T}\), \(S^{*}_{H}\), \(S^{*}_{D}\), \(E^{*}_{T}\), \(E^{*}_{H}\), \(E^{*}_{D}\), \(I^{*}_{T_{1}}\), \(I^{*}_{T_{2}}\), \(I^{*}_{H_{1}}\), \(I^{*}_{H_{2}}\), \(I^{*}_{D_{1}}\), \(I^{*}_{D_{2}}\), \(R^{*}_{T}\), \(R^{*}_{H}\) and \(R^{*}_{D}\), that minimizes \(J(u_{0}, u_{11}, u_{12}, u_{13})\) over the domain \(U_{ad}\), there exist adjoint function, \(\lambda_{i}(t)\), \(i=1,..,18\) that satisfy: \[ \dfrac{d\lambda_{i}}{dt}=-\dfrac{\partial H}{\partial x_{i}}\] where \(x_{i}=\) \(S_{T}\), \(S_{H}\), \(S_{D}\), \(E_{T}\), \(E_{H}\), \(E_{D}\), \(I_{T_{1}}\), \(I_{T_{2}}\), \(I_{H_{1}}\), \(I_{H_{2}}\), \(I_{D_{1}}\), \(I_{D_{2}}\), \(R_{T}\), \(R_{H}\), \(R_{D}\). In association with the transversality conditions \(\lambda_{l}(t_{f})=0\) for \(l=1,2,..., 18.\) Moreover, the following characterization holds
Proof. Using Pontryagin's Maximum Principle [29], the adjoint equations are obtained: \begin{align} \dfrac{d \lambda_{1}}{dt}= -\dfrac{\partial H}{\partial S_{T}}&= \alpha_{1}(\lambda_{1}-\lambda_{3})+\alpha_{2}(\lambda_{1}-\lambda_{2})+\lambda_{T}(\lambda_{1}- \lambda_{4})+\mu \lambda_{1}, \nonumber \\ \dfrac{d \lambda_{2}}{dt}= -\dfrac{\partial H}{\partial S_{H}}&= \alpha_{4}(\lambda_{2}-\lambda_{3})+\omega_{1}\lambda_{T}(\lambda_{2}- \lambda_{5})+(\mu+\mu_{11}) \lambda_{2}, \nonumber \\ \dfrac{d \lambda_{3}}{dt}= -\dfrac{\partial H}{\partial S_{D}}&= \alpha_{2}(\lambda_{3}-\lambda_{2})+\omega_{2}\lambda_{T}(\lambda_{3}- \lambda_{6})+(\mu+\mu_{12}) \lambda_{3}, \nonumber \\ \dfrac{d \lambda_{4}}{dt}= -\dfrac{\partial H}{\partial E_{T}}&=-1+\alpha_{1}(\lambda_{4}-\lambda_{6})+\alpha_{2}(\lambda_{4}-\lambda_{5})+\eta((\lambda_{4}-\lambda_{7})+\beta^{*}((\lambda_{7}-\lambda_{8})+p_{1}(\lambda_{8}-\lambda_{13})))+\mu \lambda_{4}, \nonumber \\ \dfrac{d \lambda_{5}}{dt}= -\dfrac{\partial H}{\partial E_{H}}&=-1+\alpha_{4}(\lambda_{5}-\lambda_{6})+\eta\epsilon^{*}_{1}((\lambda_{5}-\lambda_{9})+\beta^{*}((\lambda_{9}-\lambda_{10})+p_{2}(\lambda_{10}-\lambda_{14})))+(\mu+\mu_{11}) \lambda_{5}, \nonumber\\ \dfrac{d \lambda_{6}}{dt}= -\dfrac{\partial H}{\partial E_{D}}&= -1+\alpha_{2}(\lambda_{6}-\lambda_{5})+\eta\epsilon^{*}_{2}((\lambda_{6}-\lambda_{11})+\beta^{*}((\lambda_{11}-\lambda_{12})+p_{3}(\lambda_{12}-\lambda_{15})))+(\mu+\mu_{12}) \lambda_{6}, \nonumber \\ \dfrac{d \lambda_{7}}{dt}=-\dfrac{\partial H}{\partial I_{T_{1}}}&=-1+ t_{1}\alpha_{1}(\lambda_{7}-\lambda_{11})+t_{2}\alpha_{2}(\lambda_{7}-\lambda_{9})+\eta_{11}(\lambda_{7}-\lambda_{16})+(1-u_{11})l_{1}(\lambda_{7}-\lambda_{8}) \nonumber \\ \dfrac{d \lambda_{8}}{dt}=-\dfrac{\partial H}{\partial I_{T_{2}}}&=-1+(1-u_{11})\eta_{14}(\lambda_{8}-\lambda_{13})+t_{1}\alpha_{1}(\lambda_{8}-\lambda_{12})+t_{2}\alpha_{2}(\lambda_{8}-\lambda_{10})+m_{1}(\lambda_{8}-\lambda_{16}) & \nonumber \\ & + \dfrac{\alpha^{*}}{N}\big((\lambda_{1}-\lambda_{4})S_{T}+\omega_{1}S_{H}(\lambda_{2}-\lambda_{5})+\omega_{2}S_{D}(\lambda_{3}-\lambda_{6})+(1-u_{0})\beta^{'}_{1}(R_{T}(\lambda_{16}-\lambda_{4}) & \nonumber \\ &+ \omega_{1}R_{H}(\lambda_{17}-\lambda_{5})+\omega_{2}R_{D}(\lambda_{18}-\lambda_{6}))\big)+(\mu+t^{'}_{1}d_{1})\lambda_{8}, & \nonumber \\ \dfrac{d \lambda_{9}}{dt}=-\dfrac{\partial H}{\partial I_{H_{1}}}&=-1+ (1-u_{12})l_{2}(\lambda_{9}-\lambda_{10})+\eta_{12}(\lambda_{9}-\lambda_{17})+t_{3}\alpha_{4}(\lambda_{9}-\lambda_{11})& \nonumber \\ &+\dfrac{\alpha^{*}}{N}\epsilon_{1}\big((\lambda_{1}-\lambda_{4})S_{T}+\omega_{1}S_{H}(\lambda_{2}-\lambda_{5})+\omega_{2}S_{D}(\lambda_{3}-\lambda_{6})+(1-u_{0})\beta^{'}_{1}(R_{T}(\lambda_{16}-\lambda_{4}) & \nonumber \\ &+\omega_{1}R_{H}(\lambda_{17}-\lambda_{5})+\omega_{2}R_{D}(\lambda_{18}-\lambda_{6}))\big)+(\mu+\mu_{11}+d_{2})\lambda_{9},& \nonumber \\ \dfrac{d \lambda_{10}}{dt}=-\dfrac{\partial H}{\partial I_{H_{2}}}&=-1+\dfrac{\alpha^{*}}{N}\epsilon_{1}\big((\lambda_{1}-\lambda_{4})S_{T}+\omega_{1}S_{H}(\lambda_{2}-\lambda_{5})+\omega_{2}S_{D}(\lambda_{3}-\lambda_{6})+(1-u_{0})\beta^{'}_{1}(R_{T}(\lambda_{16}-\lambda_{4})& \nonumber \\& +\omega_{1}R_{H}(\lambda_{17}-\lambda_{5})+\omega_{2}R_{D}(\lambda_{18}-\lambda_{6}))\big)+t_{3}\alpha_{4}(\lambda_{10}-\lambda_{12})+m_{2}(\lambda_{10}-\lambda_{17})+(1-u_{11})\eta_{15}(\lambda_{10}& \nonumber \\ &- \lambda_{14})+ (\mu+\mu_{11}+t^{'}_{2}d_{2})\lambda_{10}, & \nonumber \\ \dfrac{d \lambda_{11}}{dt}=-\dfrac{\partial H}{\partial I_{D_{1}}}&=-1+\dfrac{\alpha^{*}}{N}\epsilon_{2}\big((\lambda_{1}-\lambda_{4})S_{T}+\omega_{1}S_{H}(\lambda_{2}-\lambda_{5})+\omega_{2}S_{D}(\lambda_{3}-\lambda_{6})+(1-u_{0})\beta^{'}_{1}(R_{T}(\lambda_{16}-\lambda_{4})& \nonumber \\ &+\omega_{1}R_{H}(\lambda_{17}-\lambda_{5})+\omega_{2}R_{D}(\lambda_{18}-\lambda_{6}))\big)+ (1-u_{13})l_{3}(\lambda_{11}-\lambda_{12})+\eta_{13}(\lambda_{11}-\lambda_{18})& \nonumber \\ &+t_{2}\alpha_{2}(\lambda_{11}-\lambda_{9})+(\mu+\mu_{12}+d_{3})\lambda_{11}, & \nonumber \end{align} \begin{align}\label{tranve1} \dfrac{d \lambda_{12}}{dt}=-\dfrac{\partial H}{\partial I_{D_{2}}}&=-1+ \dfrac{\alpha^{*}}{N}\epsilon_{2}\big((\lambda_{1}-\lambda_{4})S_{T}+\omega_{1}S_{H}(\lambda_{2}-\lambda_{5})+\omega_{2}S_{D}(\lambda_{3}-\lambda_{6})+(1-u_{0})\beta^{'}_{1}(R_{T}(\lambda_{16}-\lambda_{4})& \nonumber \\ &+\omega_{1}R_{H}(\lambda_{17}-\lambda_{5})+\omega_{2}R_{D}(\lambda_{18}-\lambda_{6}))\big)+t_{2}\alpha_{2}(\lambda_{12}-\lambda_{10})+m_{3}(\lambda_{12}-\lambda_{18}) & \nonumber \\ &+(1-u_{13})\eta_{16}(\lambda_{12}-\lambda_{15})+(\mu+\mu_{12}+t^{'}_{3}d_{3})\lambda_{12}, & \nonumber \\ \dfrac{d \lambda_{13}}{dt}=-\dfrac{\partial H}{\partial R_{T_{1}}}&=-1+ \dfrac{\alpha^{*}}{N}\big((\lambda_{1}-\lambda_{4})S_{T}+\omega_{1}S_{H}(\lambda_{2}-\lambda_{5})+\omega_{2}S_{D}(\lambda_{3}-\lambda_{6})+(1-u_{0})\beta^{'}_{1}(R_{T}(\lambda_{16}-\lambda_{4}) & \nonumber \\ &+\omega_{1}R_{H}(\lambda_{17}-\lambda_{5})+\omega_{2}R_{D}(\lambda_{18}-\lambda_{6}))\big)+\eta^{*}_{11}(\lambda_{13}-\lambda_{16})+t_{1}\alpha_{1}(\lambda_{13}-\lambda_{15})& \nonumber \\ &+t_{2}\alpha_{2}(\lambda_{13}-\lambda_{14})+(\mu+t^{*}_{1}d_{1})\lambda_{13},& \nonumber \\ \dfrac{d \lambda_{14}}{dt}=-\dfrac{\partial H}{\partial R_{H_{1}}}&=-1+ \dfrac{\alpha^{*}}{N}\epsilon_{1}\big((\lambda_{1}-\lambda_{4})S_{T}+\omega_{1}S_{H}(\lambda_{2}-\lambda_{5})+\omega_{2}S_{D}(\lambda_{3}-\lambda_{6})+(1-u_{0})\beta^{'}_{1}(R_{T}(\lambda_{16}-\lambda_{4})& \nonumber \\ &+\omega_{1}R_{H}(\lambda_{17}-\lambda_{5})+\omega_{2}R_{D}(\lambda_{18}-\lambda_{6}))\big)+ \eta^{*}_{12}(\lambda_{14}-\lambda_{17})+t_{3}\alpha_{4}(\lambda_{14}-\lambda_{15})& \nonumber \\ &+(\mu+\mu_{11}+t^{*}_{2}d_{2})\lambda_{14},& \nonumber \\ \dfrac{d \lambda_{15}}{dt}=-\dfrac{\partial H}{\partial R_{D_{1}}}&=-1+ \dfrac{\alpha^{*}}{N}\epsilon_{2}\big((\lambda_{1}-\lambda_{4})S_{T}+\omega_{1}S_{H}(\lambda_{2}-\lambda_{5})+\omega_{2}S_{D}(\lambda_{3}-\lambda_{6})+(1-u_{0})\beta^{'}_{1}(R_{T}(\lambda_{16}-\lambda_{4})& \nonumber \\ &+\omega_{1}R_{H}(\lambda_{17}-\lambda_{5})+\omega_{2}R_{D}(\lambda_{18}-\lambda_{6}))\big)+\eta^{*}_{13}(\lambda_{15}-\lambda_{18})+t_{2}\alpha_{2}(\lambda_{15}-\lambda_{14})& \nonumber \\ &+(\mu+\mu_{12}+t^{*}_{3}d_{3})\lambda_{15},& \nonumber \\ \dfrac{d \lambda_{16}}{dt}=-\dfrac{\partial H}{\partial R_{T}}&=(1-u_{0})\beta^{'}_{1}\lambda_{T}(\lambda_{16}-\lambda_{4})+\alpha_{1}(\lambda_{16}-\lambda_{18})+\alpha_{2}(\lambda_{16}-\lambda_{17})+\mu \lambda_{16}, & \nonumber \\ \dfrac{d \lambda_{17}}{dt}=-\dfrac{\partial H}{\partial R_{H}}&=(1-u_{0})\beta^{'}_{1}\lambda_{T}\omega_{1}(\lambda_{17}-\lambda_{5})+\alpha_{4}(\lambda_{17}-\lambda_{18})+(\mu+\mu_{11}) \lambda_{17},& \nonumber \\ \dfrac{d \lambda_{18}}{dt}=-\dfrac{\partial H}{\partial R_{D}}&=(1-u_{0})\beta^{'}_{1}\lambda_{T}\omega_{2}(\lambda_{18}-\lambda_{6})+\alpha_{2}(\lambda_{18}-\lambda_{17})+(\mu+\mu_{12}) \lambda_{18}. & \end{align} Optimality is when the equations \(\dfrac{\partial H}{\partial u_{j}}=0\) at \(u^{*}_{0}\), \(u^{*}_{1i}\) for \(i=1, 2, 3\). Then, \[\dfrac{\partial H}{\partial u_{0}}=B_{0}u_{0}+\beta^{'}_{1}\lambda_{T}\big((\lambda_{16}-\lambda_{4})R_{T}+\omega_{1}(\lambda_{17}-\lambda_{5})R_{H}+\omega_{2}(\lambda_{18}-\lambda_{6})R_{D}\big)=0\,,\] which implies that \[u^{*}_{0}=\dfrac{\beta^{'}_{1}\lambda_{T}\big((\lambda_{4}-\lambda_{16})R_{T}+\omega_{1}(\lambda_{5}-\lambda_{17})R_{H}+\omega_{1}(\lambda_{6}-\lambda_{18})R_{D}\big)}{B_{0}}\,,\] on the set \(\lbrace t: 0< u^{*}_{0}(t)< 1 \rbrace\). \[\dfrac{\partial H}{\partial u_{11}}=(B_{1}+B_{4})u_{11}+l_{1}I_{T_{1}}(\lambda_{7}-\lambda_{8})+\eta_{14}I_{T_{2}}(\lambda_{8}-\lambda_{13})R_{D}=0\,,\] which implies that \[u^{*}_{11}=\dfrac{l_{1}I_{T_{1}}(\lambda_{8}-\lambda_{7})+\eta_{14}I_{T_{2}}(\lambda_{13}-\lambda_{8})}{B_{1}+B_{4}}\,,\] on the set \(\lbrace t: 0< u^{*}_{11}(t)< 1 \rbrace\).
Analogously, for the optimal control \(u^{*}_{12}\), we have
\[\dfrac{\partial H}{\partial u_{12}}=(B_{2}+B_{5})u_{12}+l_{2}I_{H_{1}}(\lambda_{9}-\lambda_{10})+\eta_{15}I_{H_{2}}(\lambda_{10}-\lambda_{14})R_{H}=0.\] Therefore, \[u^{*}_{12}= \dfrac{l_{2}I_{H_{1}}(\lambda_{10}-\lambda_{9})+\eta_{15}I_{H_{2}}(\lambda_{14}-\lambda_{10})}{B_{2}+B_{5}}\,,\] on the set \(\lbrace t: 0< u^{*}_{12}(t)< 1 \rbrace\). For the control \(u^{*}_{13}\), we obtained \[\dfrac{\partial H}{\partial u_{13}}=(B_{3}+B_{6})u_{13}+l_{3}I_{D_{1}}(\lambda_{11}-\lambda_{12})+\eta_{16}I_{D_{2}}(\lambda_{12}-\lambda_{15})=0,\] and this implies that \[u^{*}_{13}= \dfrac{l_{3}I_{D_{1}}(\lambda_{12}-\lambda_{11})+\eta_{16}I_{D_{2}}(\lambda_{15}-\lambda_{12})}{B_{3}+B_{6}}\,,\] on the control set \(\lbrace t: 0< u^{*}_{13}(t)< 1\rbrace \).Note that the optimality conditions only hold on the interior of the control set.
4. Numerical Results
The aim of this section is to simulate the application of the controls in the population. First, we solve the optimality system numerically using an iterative method based on the fourth-order Runge-Kutta. We use the forward-backward sweep method for finding the solution of the optimality system which has the state Eq. (1), adjoint Eq. (11), control characterizations (10), and initial/final condition (initial and transversality conditions). The method starts with initial values for the optimal control and we solve the state system forward in time using the Runge-Kutta method of the fourth-order. Following, we solve the adjoint equation backward in time with Runge-Kutta of the fourth-order using the state variables, initial control guesses, and transversality conditions. The controls \(u_{0}(t)\), \(u_{11}(t)\), \(u_{12}(t)\) and \(u_{13}(t)\) are updated and used to solve the state and adjoint system respectively. This iterative process continues until that current state, adjoint, and control values converge [19,30].For the numerical simulations, we use a set of parameters extracted from [31,32,33,34,35,36,37,38,39,40,41] with purposes illustrative and to support the analytical results, see Table 2.
The initial conditions for the TB-Only and TB-Diabetes sub-populations are taken from [31], and the values for th1e sub-population of HIV/AIDS are assumed and do not represent a specific demographic area, but fall within the range of actual achievable data, see Table 2. The values of the parameters and initial conditions that are assumed are discussed and validated with the specialists. We assume that \(B_{0}=200\), \(B_{1}=50\), \(B_{2}=150\), \(B_{3}=75\), \(B_{4}=100\), \(B_{5}=250\) and \(B_{3}=150\).
We assume that always apply control in the HIV/AIDS sub-population because tuberculosis is classified as an opportunistic disease and HIV/AIDS cases are monitored by the use of antiretroviral therapy. Also, reinfection or reactivation of TB is always controlled in all strategies because of its impact on treatment resistance. Then, our control strategies are defined as the combination of efforts and are defined as:
Strategy I. We activate all controls (\(u_{0}(t), u_{11}(t), u_{12}(t)>0, u_{13}(t)>0\)).
Strategy II. Combination of \(u_{0}(t)\), \(u_{11}(t)\), \(u_{12}(t)\) while setting \(u_{13}(t)=0\) (\(u_{0}(t), u_{11}(t)>0, u_{12}(t)>0\) and \(u_{13}(t)=0\)).
Strategy III. Combination of \(u_{0}(t)\), \(u_{12}(t)\), \(u_{13}(t)\) while setting \(u_{11}(t)=0\) (\(u_{0}(t),u_{12}(t),u_{13}(t)>0\) and \(u_{11}(t)=0\)).
We are going to study how we start the control process, with high efficient control (type I) and with minimum value (type II). Figure 2 shows the profiles of the resistance controls (\(u_{11}(t), u_{12}(t), u_{13}(t)\)) over time for the different strategies and control types.Strategy I. In this strategy all controls are active (\(u_{0}(t)>0\), \(u_{11}(t)>0\), \(u_{12}(t)>0\) and \(u_{13}(t)>0\)). In other words, reinfection or reactivation and resistance are controlled. We show the behavior of the controls over time, see Figure 2a for control type I and see Figure 2b for control type II. It is observed that when this control strategy is implemented, there is a significant decrease in the number of TB resistant compared with the model without control, see Figure 3. In the case of MDR-TB in the different sub-populations before the study year, a decrease in the number of reported cases was observed, see Figures 3a-3c. In the case of XR-TB, the reduction in the number of cases will occur over a longer period of time, but this reduction is significant, mainly in XDR-TB diabetics, which has a strong incidence in the dynamics, see Figure 3d-3f. In the case of MDR-TB and XDR-TB in the TB-HIV/AIDS sub-population, the control manages to avoid the growth of the number of cases because in the dynamics these compartments tend to decrease initially and then grow. This strategy takes advantage of the decrease in the number of cases by preventing future growth. The type I control showed better results so it is recommended to start with higher efficacy and this evolves showing better results.
Strategy II. Here we activate the controls \(u_{0}(t)>0\), \(u_{11}(t)>0\) and \(u_{12}(t)>0\) and \(u_{13}(t)=0\), this means that we control resistance in the TB-HIV/AIDS and TB-Only sub-populations and reinfection or reactivation TB in the full model. The behavior of the controls is shown in Figure 2c-2d.
Table 2. Values of variables and parameters used in the model (1).
Variables | Value | Variables | Value | Variables | Value |
---|---|---|---|---|---|
\(S_{T}(0) \) | 8741400 | \(S_{H}(0) \) | 111000 | \(S_{D}(0) \) | 200000 |
\(E_{T}(0) \) | 565600 | \(E_{H}(0) \) | 5000 | \(E_{D}(0) \) | 8500 |
\(I_{T_{1}}(0) \) | 20000 | \(I_{H_{1}}(0) \) | 1400 | \(I_{D_{1}}(0) \) | 1800 |
\(I_{T_{2}}(0) \) | 1300 | \(I_{H_{2}}(0) \) | 400 | \(I_{D_{2}}(0) \) | 550 |
\(R_{T_{1}}(0) \) | 700 | \(R_{H_{1}}(0) \) | 210 | \(R_{D_{1}}(0) \) | 250 |
\(R_{T}(0) \) | 8800 | \(R_{H}(0) \) | 500 | \(R_{D}(0) \) | 300 |
Parameters | Value | Reference | Parameters | Value | Reference |
\(M_{1}, \) \(M_{2}, \) \(M_{3} \) | \(667685, 10000, 50000 \) | [31], Assumed, Assumed | \(\alpha^{*} \) | \( 9.5 \) | [31, 32, 33] |
\(\alpha_{1} \), \(\alpha_{2} \) | \(0.0075 \), \(0.009 \) | Assumed, [31,32] | \(\omega_{1}, \) \(\omega_{2} \) | \(1.22 ,1.10 \) | [31, 32, 34], assumed |
\(\alpha_{4} \) | 17.3 (per thousand people per year) | [35] | \(\epsilon_{1}, \epsilon_{2} \) | 1.3, 1.1 | Assumed, [31, 32] |
\(\mu, \mu_{11}, \mu_{12} \) | \(1/53.5, 0.045,0.03 \) | [31, 32, 34], Assumed | \(\eta \), \(\beta^{*} \) | 0.5, 0.04 | [31, 32, 34,36, 37] |
\(d_{1}, \) \(d_{2}, \) \(d_{3} \) | \(0.275 \), \(0.33 \), \(1.5*d_{1} \) | [31, 32, 34] | \(\epsilon^{*}_{1}, \epsilon^{*}_{2} \) | 1.3, 1.1 | [34], Assumed |
\( t^{*}_{1}, t^{*}_{2}, t^{*}_{3} \) | 1.01, 1.02, 1.01 | Assumed | \(t^{'}_{1}, t^{'}_{2}, t^{'}_{3} \) | 1, 1.01, 1 | Assumed |
\(\beta^{'}_{1} \) | 0.9 | [34] | \(l_{1}, \) \(l_{2}, \) \(l_{3} \) | 0.0018, 0.0022, 0.0048 | [33, 38, 39, 40], Assumed |
\(m_{1}, \) \(m_{2}, \) \(m_{3} \) | \(0.6266 \), \(0.45 \), \(0.4054 \) | [31, 32], Assumed | \(\eta_{14}, \) \(\eta_{15}, \) \(\eta_{16} \) | 0.013, 0.022, 0.006 | [33, 38, 39, 40], Assumed |
\(\eta_{11}, \) \(\eta_{12}, \) \(\eta_{13} \) | \(0.7372 \), \(0.55 \), \(0.7372 \) | [31, 32], Assumed | \(p_{1}, p_{2}, p_{3} \) | 0.00225,0.0035,0.0041 | [36, 37, 41], Assumed |
\(\eta_{11}^{*}, \) \(\eta_{12}^{*}, \) \(\eta_{13}^{*} \) | \(0.4006 \), \(0.255 \), \(0.3317 \) | [31, 32], Assumed | \( t_{1}, t_{2}, t_{3} \) | 1.01, 1.01, 1.01 | Assumed |
Strategy III. This strategy does not control resistance in the TB-Only sub-population. As in the previous strategies, the objective of reducing resistance in the dynamics is met. Controls in XDR-TB HIV/AIDS and diabetes reduced the number of cases and asymptotic behavior was maintained, but the results were better than strategy II for the different types of controls. This strategy also failed to take advantage of the decrease in the number of cases, reducing the number of cases but not avoiding future asymptotic growth, see Figures 5a, 5e and 5f. Here too, type I controls achieved better results.
In general, all strategies and types of controls met the objective of reducing the number of cases of MDR-TB and XDR-TB. The most efficient strategy was the strategy I with type I control. In addition to significantly reducing the number of cases in all resistance compartments and also takes advantage of the decrease in dynamics and prevents the future growth of cases. In all strategies, type I control showed better results, so it is recommended to start with low control. In [25] the numerical results show the need to reduce XDR-TB in diabetics due to the growth that occurs in this sub-population, so strategy II does not meet significantly this objective. Strategy II is not recommended because it fails to significantly reduce resistance in diabetics and diabetes is a risk factor for adherence to TB treatment.
5. Conclusions
In this work, we have studied the problem of control in patients to achieve greater adherence to treatment taking into account the influence of HIV/AIDS and diabetes and thus avoid MDR-TB and XDR-TB. We present the stability at the disease-free equilibrium point related to the basic reproduction number for the sub-models and proved the global stability of this point for the full model (1). The controls are defined as \(u_{0}\), \(u_{11}\), \(u_{12}\) and \(u_{13}\) and are based on avoiding reinfection or reactivation of the bacteria and on differentiated care and follow-up in cases who are neither HIV+ nor diabetics, HIV/AIDS, and diabetics. The optimal control theory for the model is derived analytically by applying Pontryagin’s maximum principle and we demonstrate the existence of optimal control. For the computational simulations, we used a fourth-order Runge-Kutta forward/backward scheme. We experimented in different scenarios built with different combinations of the controls. We present the results of the resistance compartments (\(I_{T_{2}}\), \(I_{H_{2}}\), \(I_{D_{2}}\), \(R_{T_{1}}\), \(R_{H_{1}}\) and \(R_{D_{1}}\)). We concluded that all variants of strategies with the different types of controls met the objective of reducing the number of resistant cases. The strategy that obtained the best results was the strategy I (activating all controls) with type I controls (starting with the highest controls). Recommend keeping all sub-populations under control and starting with maximum control. However, if we have to use only three control, we recommended using strategy III, because all the resistance compartments and mainly the diabetic XDR-TB are reduced compared with strategy II. We do not recommend the use of strategy II, since one of the main factors of resistance to TB treatment is diabetes and this strategy did not manage to reduce significantly the number of resistant cases to TB treatment in diabetics. The results of this work help those responsible for health systems to make decisions for the efficacy of tuberculosis treatment, taking into account the influence of HIV/AIDS and diabetes. In future works, we propose to experiment in a real scenario and use differential inclusions for the control system.Figure 2. The profiles of the controls associated with resistance in the different strategies and for the different types of controls.
Figure 3. Comparison in the resistance compartments between the types of controls for strategy I.
Figure 4. Comparison in the resistance compartments between the types of controls for strategy II.
Figure 5. Comparison in the resistance compartments between the types of controls for strategy III.
Author Contributions:
All authors contributed equally in this paper. All authors read and approved final version of this paper.Conflicts of Interest:
The author declares no conflict of interest.Data Availability:
All data required for this research is included within this paper.Funding Information:
No funding is available for this research.References
- Delogu, G., Sali, M., & Fadda, G. (2013). The biology of mycobacterium tuberculosis infection. Mediterranean Journal of Hematology and Infectious Diseases, 5(1), Article ID: e2013070. https://doi.org/10.4084/mjhid.2013.070. [Google Scholor]
- World Health Organization (WHO): Global Tuberculosis Report. (2017). https://www.who.int/tb/publications/global_report/gtbr2017_main_text.pdf?ua=1. Accessed on 10 January 2019. [Google Scholor]
- World Health Organization (WHO). (2006). Extensively drug-resistant tuberculosis (XDR-TB): recommendations for prevention and control. Weekly Epidemiological Record= Relevé Épidémiológique Hebdomadaire, 81(45), 430-432. [Google Scholor]
- Gandhi, N. R., Moll, A., Sturm, A. W., Pawinski, R., Govender, T., Lalloo, U., ... & Friedland, G. (2006). Extensively drug-resistant tuberculosis as a cause of death in patients co-infected with tuberculosis and HIV in a rural area of South Africa. The Lancet, 368(9547), 1575-1580. [Google Scholor]
- Niazi, A. K., & Kalra, S. (2012). Diabetes and tuberculosis: a review of the role of optimal glycemic control. Journal of Diabetes & Metabolic Disorders, 11, Article No 28. https://doi.org/10.1186/2251-6581-11-28. [Google Scholor]
- Stevenson, C. R., Critchley, J. A., Forouhi, N. G., Roglic, G., Williams, B. G., Dye, C., & Unwin, N. C. (2007). Diabetes and the risk of tuberculosis: a neglected threat to public health?. Chronic Illness, 3(3), 228-245. [Google Scholor]
- Jeon, C. Y., & Murray, M. B. (2008). Diabetes mellitus increases the risk of active tuberculosis: A systematic review of 13 observational studies. PLoS Med., 5(7), Article ID: e152. https://doi.org/10.1371/journal.pmed.0050152. [Google Scholor]
- Baghaei, P., Marjani, M., Javanmard, P., Tabarsi, P., & Masjedi, M. R. (2013). Diabetes mellitus and tuberculosis facts and controversies. Journal of Diabetes & Metabolic Disorders, 12, Article No 58. https://doi.org/10.1186/2251-6581-12-58. [Google Scholor]
- Basoglu, O. K., Bacakoglu, F., Cok, G., Saymer, A., & Ates, M. (1999). The oral glucose tolerance test in patients with respiratory infections. Monaldi Archives for Chest Disease, 54, 307-310. [Google Scholor]
- Mathema, B., Kurepina, N. E., Bifani, P. J., & Kreiswirth, B. N. (2006). Molecular epidemiology of tuberculosis: current insights. Clinical Microbiology Reviews, 19(4), 658-685. [Google Scholor]
- McIvor, A., Koornhof, H., & Kana, B. D. (2017). Relapse, re-infection and mixed infections in tuberculosis disease. Pathogens and Disease, 75(3), Article ID: ftx020. https://doi.org/10.1093/femspd/ftx020. [Google Scholor]
- Kim, S., Aurelio, A., & Jung, E. (2018). Mathematical model and intervention strategies for mitigating tuberculosis in the Philippines. Journal of Theoretical Biology, 443, 100-112. [Google Scholor]
- Silva, C. J., & Torres, D. F. (2013). Optimal control for a tuberculosis model with reinfection and post-exposure interventions. Mathematical Biosciences, 244(2), 154-164. [Google Scholor]
- Jung, E., Lenhart, S., & Feng, Z. (2002). Optimal control of treatments in a two-strain tuberculosis model. Discrete & Continuous Dynamical Systems-B, 2(4), 473–482. [Google Scholor]
- Silva, C. J., Maurer, H., & Torres, D. F. M. (2017). Optimal control of a tuberculosis model with state and control delays. Mathematical Biosciences and Engineering, 14(2): 321-327. [Google Scholor]
- Silva, C. J., & Torres, D. F. (2013). Optimal control for a tuberculosis model with reinfection and post-exposure interventions. Mathematical Biosciences, 244(2), 154-164. [Google Scholor]
- Tahir, M., Shah, S. I. A., & Zaman, G. (2019). Prevention strategy for superinfection mathematical model tuberculosis and HIV associated with AIDS. Cogent Mathematics & Statistics, 6(1), Article ID: 1637166. https://doi.org/10.1080/25742558.2019.1637166. [Google Scholor]
- Awoke, T. D., & Kassa, S. M. (2018). Optimal control strategy for TB-HIV/AIDS Co-Infection Model in the Presence of Behaviour Modification. Processes, 6(5), 48-73. [Google Scholor]
- Agusto, F. B., & Adekunle, A. I. (2014). Optimal control of a two-strain tuberculosis-HIV/AIDS co-infection model. Biosystems, 119, 20-44. [Google Scholor]
- Silva, C. J., & Torres, D. F. M. (2015). A TB-HIV/AIDS co-infection model and optimal control treatment. Discrete & Continuous Dynamical System Serie B, 35(9), 4639-4663. [Google Scholor]
- Fatmawati & Tasman, H. (2016). An Optimal Treatment Control of TB-HIV Co-infection. International Journal of Mathematics and Mathematical Sciences, 2016, Article ID 8261208. https://doi.org/10.1155/2016/8261208. [Google Scholor]
- Tanvi, R. Aggarwal. (2020). Optimal Control Analysis of HIV-TB Co-infection Model. In: Mondaini R. (eds) Trends in Biomathematics: Modeling Cells, Flows, Epidemics, and the Environment. BIOMAT 2019. Springer, Cham. https://doi.org/10.1007/978-3-030-46306-9_17. [Google Scholor]
- Kouidere, A., Labzai, A., Ferjouchia, H., Balatif, O., & Rachik, M. (2020). A new mathematical modeling with optimal control strategy for the dynamics of population of diabetics and its complications with effect of behavioral factors. Journal of Applied Mathematics, 2020, Article ID 1943410. https://doi.org/10.1155/2020/1943410. [Google Scholor]
- Chávez, I. Y. S., Morales-Menéndez, R., & Chapa, S. O. M. (2009). Glucose optimal control system in diabetes treatment. Applied Mathematics and Computation, 209, 19–30. [Google Scholor]
- Moya, E. D., Pietrus, A., & Oliva, S. M. (2021). A mathematical model for the study of effectiveness in therapy in Tuberculosis taking into account associated diseases. Contemporary Mathematics, 2, 77-102. [Google Scholor]
- Driessche, P. V., & Watmough, J. (2002). Reproduction numbers and sub-threshold endemic equilibria for compartmental models of disease transmission. Mathematical Biosciences, 180(1-2), 29-48. [Google Scholor]
- Castillo-Chavez, C., Feng, Z., & Huang, W. (2002). On the computation of \(\Re_{0}\) and its role on global stability. In: Mathematical Approaches for Emerging and Reemerging Infectious Diseases: An Introduction, Springer-Verlag, New York, 229-250. [Google Scholor]
- Fleming, W. H., & Rishel, R. W. (1975). Deterministic and Stochastic Optimal Control. Springer Verlag New York. [Google Scholor]
- Pontryaging, L., Boltyankij, V., Gamkrelvdze, R. & Mishchenko, E. (1962). The Mathematical Theory of Optimal Processes. Jonh Wiley and Sons, New Yor, NY, USA. [Google Scholor]
- Lenhart, S., & Workman, J. T. (2007). Optimal Control Applied to Biological Models. Chapman and Hall, Boca Raton. [Google Scholor]
- Carvalho, A. R., & Pinto, C. M. (2018). Non-integer order analysis of the impact of diabetes and resistant strains in a model for TB infection. Communications in Nonlinear Science and Numerical Simulation, 61, 104-126. [Google Scholor]
- Moualeu, D. O., Bowong, S., Tewa, J. J., & Emviedu, Y. (2011). Analysis of the impact of diabetes on the dynamical transmission of tuberculosis. Mathematical Modelling of Natural Phenomena, 7(3), 117-146. [Google Scholor]
- Agusto, F. B., Cook, J., Shelton, P. D., & Wickers, M. G. (2015). Mathematical model of MDR-TB and XDR-TB with isolation and lost to follow-up. Abstract and Applied Analysis, 2015, Article ID 828461. https://doi.org/10.1155/2015/828461. [Google Scholor]
- Silva, C., & Torres, D. F. M. (2015). A TB-HIV/AIDS co-infection model and optimal control treatment. Discrete & Continuous Dynamical Systems, 35(9), 4639-4663. [Google Scholor]
- Cassetone, A. J. F. Advisor: Segurado, A. A. C., & co-advisor: Abe, J. M. (2019). Alteraçóes metabólicas associadas ao uso de medicamento antirretroviral em pessoas vivendo com HIV/AIDS: caracterizaç ào e desenvolvimento de algoritmos inteligentes aplicados à sua identificaç ào e previsào. Tese de Doutorado, USP. https://doi.org/10.11606/T.5.2017.tde-14122017-112914. [Google Scholor]
- Castillo-Chavez, C., & Feng, Z. (1997). To treat or not to treat: The case of tuberculosis. Journal of Mathematical Biology, 35(6), 629-56. [Google Scholor]
- Jung, E., Lenhart, S., & Feng, Z. (2002). Optimal control of treatments in a two-strain tuberculosis model. Discrete & Continuous Dynamical Systems-B, 2(4), 473-482. [Google Scholor]
- Hickson, R. I., Mercer, G. N., & Lokuge, K. M. (2012). A metapopulation model of tuberculosis transmission with a case study from high to low burden areas. PLoS One, 7(4), Article ID: e34411. https://doi.org/10.1371/journal.pone.0034411.[Google Scholor]
- Blower, S. M., Mclean, A. R., Porco, T. C., Small, P. M., Hopewell, P. C., Sanchez, M. A., & Moss, A. R. (1995). The intrinsic transmission dynamics of tuberculosis epidemics. Nature Medicine, 1(8), 815-821.[Google Scholor]
- Cohen, T., Colijin, C., Finklea, B., & Murray, M. (2007). Exogeneus re-infection and the dynamics of tuberculosis epidemics: local effects in a network model of transmission. Journal of the Royal Society Interface, 4(14), 523-531. [Google Scholor]
- Liu, Y., Sun, Z., Sun, G., Zhong, Q., Jiang, L., Zhou, L., ... & Jia, Z. (2011). Modeling transmission of tuberculosis with MDR and undetected cases. Discrete Dynamics in Nature and Society, 2011, Article ID: 296905. https://doi.org/10.1155/2011/296905. [Google Scholor]